Working Smarter
Episode 4: Babak Hodjat on building smarter, more helpful AI
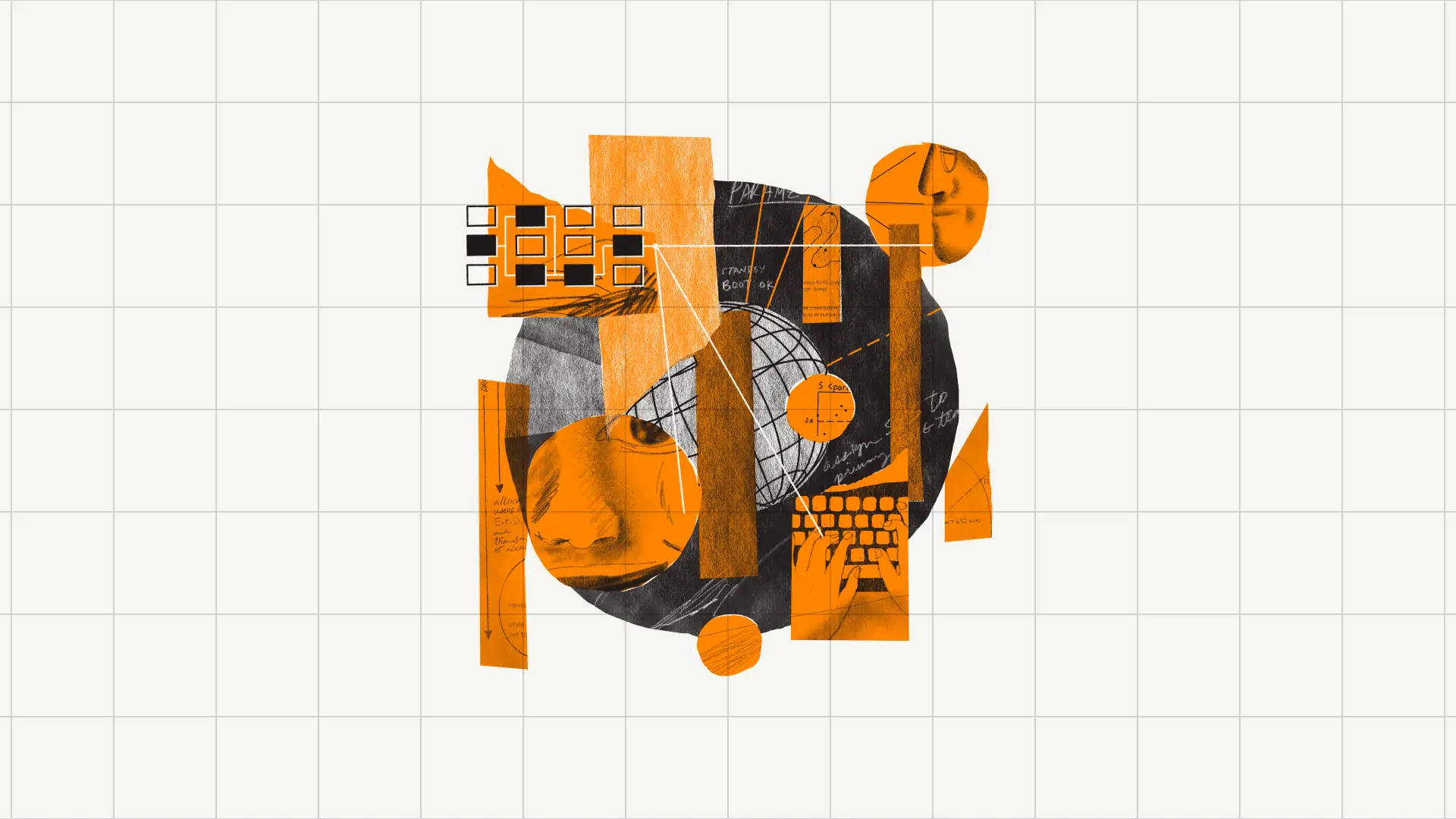
For our fourth episode of Working Smarter we’re talking to Babak Hodjat, the chief technology officer for AI research at Cognizant. If you’ve ever used Apple’s smart assistant Siri, then you’re almost certainly familiar with his work.
Hodjat has been doing AI research for nearly four decades, and if there’s one thread that runs through his career, it’s how to make working with digital agents feel as natural or effortless as working with a colleague. At Cognizant—an IT services and consulting firm—Hodjat’s team puts this work into practice by helping other companies integrate AI tools into their workflows.
Hear Hodjat discuss why it’s still so hard for machines to understand exactly what we want them to do, the problems he’s helping his customers solve, and how the latest generation of workplace assistants can help us make better decisions and improve the way we do our jobs.
Show notes:
- Learn more about Cognizant's Advanced AI Lab
- Links to Hodjat’s research
- The Perceptive Assistant that Learns (PAL)
- The Cognitive Assistant that Learns and Organizes (CALO)
~ ~ ~
Working Smarter is a new podcast from Dropbox about how AI is changing the way we work and get stuff done.
You can listen to more episodes of Working Smarter on Apple Podcasts, Spotify, YouTube Music, Amazon Music, or wherever you get your podcasts. To read more stories and past interviews, visit workingsmarter.ai
This show would not be possible without the talented team at Cosmic Standard, namely: our producers Samiah Adams and Aja Simpson, technical director Jacob Winik, and executive producer Eliza Smith. Special thanks to Benjy Baptiste for production assistance, our marketing and PR consultant Meggan Ellingboe, and our illustrators, Fanny Luor and Justin Tran. Our theme song was created by Doug Stuart. Working Smarter is hosted by Matthew Braga.
Thanks for listening!
Full episode transcript
Growing up, I earned a reputation as the guy who was “good with computers.” I’d update my family’s PC and keep it virus-free. I’d help my friends burn CDs and download MP3s. I’d use my supposedly arcane knowledge to install software for my teachers, and edit the videos I made with friends at school.
As an adult, I sort of assumed we’d have figured all this stuff out by now. I thought I’d be obsolete! But the more things change, the more they stay the same. I still help my in-laws with their TV and make sure my mom’s computer is always backed up (with Dropbox, of course). Whenever my best friend’s phone stops working, you can probably guess who she calls.
Nothing feels as seamless or easy as it should be. Especially when it comes to the way we use technology for work. But I don’t think it’s our fault. If our apps and devices are so powerful, why aren’t they better at understanding—anticipating, even—the things we want to do in whatever way makes the most sense to us?
I’m your host Matthew Braga—and today I’ll be talking to Babak Hodjat, the chief technology officer for AI research at Cognizant, an IT services and consulting firm.
Babak’s team helps other companies integrate AI tools into their workflows. It’s work he’s been doing, if you can believe it, for nearly four decades. And if there’s one thread that runs through Babak’s career, it’s how to make working with technology feel as natural or effortless as working with a colleague—or the “good with computers” person in your life. Easier said than done, right? But with recent advances in AI, we might actually have a shot.
Oh, and trust me on this: even if you don’t know Babak, you’re almost certainly familiar with his work. That’s coming up next on this episode of Working Smarter.
~ ~ ~
Babak, thank you so much for joing us.
Thank you for having me.
To start off, who are you and what you do?
I am the CTO for AI for Cognizant, which means I lead an AI R&D team here in downtown San Francisco. My background is in AI. I have a PhD in AI and I got into AI in the late ‘80s. I started several companies, one of them led to Siri, so I was the main inventor of the natural language technology behind Siri. Although I was not officially part of Siri, my team started Siri. Then, I started Sentient Technologies where we worked on distributed AI, and then we joined forces with Cognizant. In a roundabout way, I feel like I'm back to natural language again.
Well, we’re going to dig deeper into a bunch of that, but I think the first thing I wanted to ask you—and I think it’s a good place to start—is, as you say, you’ve been described as the co-creator and the co-inventor of the technology that eventually became Siri. I was wondering if you could explain the story behind that.
I was working on agent-based AI in the late 90s and was looking for an application for it because I felt like it was very powerful. A friend misunderstood what I was doing when I said "agent-based” and thought agents are these representatives of humans with whom you can speak natural language and who would understand and then go do some stuff on your behalf. When I told him that’s not what I meant, and that natural language is very, very hard, he challenged me. He said, "Well, if you think your AI is powerful, why don’t you apply multi-agent systems to natural language?"
I took that challenge on, worked on it, and came up with an approach that was very different than how people did natural language. They used grammar-based and language-based approaches before. This one starts from the ontology of the domain that you’re talking about, which is very different. That led to Dejima, which was my first startup in ‘97, ‘98. At Dejima, we worked on a project that DARPA was running called the CALO project, which was done through SRI.
The company Siri was born out of that CALO project at SRI. Our VP of engineering, architects, and a lot of the folks that were at Dejima ended up working on Siri, and they did adopt the core natural language technique and technology that we had come up with.
I'm curious, your friend had one idea of agents and what agents meant. What were you thinking about when you thought of the word agents? What did that mean in your context?
So, AI started off being this quest for building an all-intelligent system. And in the late ‘80s and ‘90s, AI scientists realized that that's too big a problem to tackle. So, what if we actually simplify the problem? In a simplified world, use an AI system to operate in that world rather than the outside world with all its complexity. That is what they refer to as an agent. A multi-agent system came out of the fact that if I have an agent on the World Wide Web, how would it interact with another agent? How would they communicate? Would they be collaborative or competing? This multi-agent concept started there.
What I was working on was imagining many "idiot savant" agents that have very simplistic worlds they operate in. Can you get them to solve larger problems just by virtue of the emergent behavior that comes out of them trying to survive in an environment? While it was still called agents, in some ways it was more about simplifying AI.
What my friend misunderstood was, he was actually at a bar, and this lady, this old mama-san at the bar in Japan, was trying to get the tennis game. And she tried with the remote and everything and couldn't get it. So she turned to her son and said, "Get me the tennis." The complexity of "get me the tennis" involved finding the right channel, operating the remote, and turning on the TV. The agent, in this case, would handle all those tasks based on her intent. It's worlds apart from what I was working on, and I can tell you, natural language is hard. It's still hard.
Everything you're describing—this idea of splitting the problem into all these different agents, they handle different tasks, they do different things, and you have to figure out how to wrangle them all and make them talk to one another—does it feel like we’ve come full circle again into this present moment, given the things that people are building? Because it seems like people are trying to solve a similar problem now, building tools that can interact with all of your different apps, your different devices, different contexts, different data sources.
We have come full circle but the reasons why we're now operating agents is very, very different. It's actually completely the opposite. So back then it was because our AI wasn't powerful enough. So we had to simplify the environment they operated in. That was the agent.
Today, our AI systems are powerful—in fact, so powerful—that we have to contain them. They're so robust. This agent can take any different persona. You can tell it that it's an expert in field one or an expert in field two or an ordinary user or a hacker or whatever. And depending on what persona you give it, it'll have a different behavior.
For us to be able to utilize this AI, we have to actually limit its operation to the workflow that we're interested in. And that's brought us full circle back to, "okay, we have to think about these large language models as agents when we plug them into a workflow."
So yeah, different reasons, but the end result is the same. And in fact, I think we are moving towards a multi-agent world because now, as we set up these workflows, we want different agents responsible and being experts in different parts of the workflow to work together. But the world is much simpler. Like we can program these agents using natural language. We can actually tell them what we expect from them. They can talk to each other in natural language. And that is worlds apart from the multi-agent systems that we had back then. We had to define inter-agent communication languages that were very elaborate and were hiding certain information and revealing certain other information and so forth. And you don't need to do that.
Well, and to your point, we've obviously gotten a lot better at doing this kind of thing today, but there are still challenges with making our technology understand what we want it to do. It still seems like there's a bit of a way to go. Why is it so hard for our machines to understand the intent behind what we want them to do in a natural way, rather than us having to contort ourselves to what our machines expect from us?
There's several reasons, one being that currently the state of the art is a very question-answering kind of state of the art, and the context that these large language models have is limited to how you express yourself in natural language. Natural language by nature is ambiguous and could be terse and takes a lot for granted as far as the context is concerned. And these large language models have nothing to go by other than the exact words that you tell them.
You just nodded. That nod tells me that you kind of agree with me or you appreciate the point that I'm making. They don't see that. So of course, we're moving beyond that. We're trying to make them multimodal. We're trying to make them more reactive to other cues. So that will help. But that's one limitation that we have. The other limitation that we have is the fact that these systems are pre-trained.
The PT in GPT is pre-trained, which means that their world model is fixed. And because they're very, very robust, they can be pushed to view the input and behave differently, depending on what we ask them and how we set them up. But unlike humans or other higher order intelligent animals, they don't learn as they go. They're not embodied, and that embodiment really helps us situate ourselves in a context and understand what's going on.
In spite of all of this, I must still say that, as humans, we misunderstand each other all the time. I mean, that's just a byproduct of how our languages have evolved. So you can't blame machines for misunderstanding. We're no longer programming them in a non-ambiguous programming language. We're literally using the same language, the same operating system we use ourselves in our community. So some level of misunderstanding is just par for the course.
Earlier you mentioned the CALO project that you and some colleagues worked on that helped to lay the groundwork for Siri. And I was looking back at some articles that were written around the time about some of that work—specifically about the Perceptive Assistant that Learns or PAL that your company was working on for DARPA. At the time, in these articles, PAL was described as an office assistant, something that could set up meetings, answer emails. It's a lot of the same stuff that people seem to be talking about using AI and specifically LLMs for today. And I'm wondering what you make of that. I mean, is that notable that the path forward almost two decades ago was sort of similar to what people are identifying now?
Yeah, I think it's the case that that is still a hard problem and has not been solved. I think it was year two or year three of the CALO project when we actually set up a system where you would pick up the phone and you would tell CALO, basically, that you wanted to set up a meeting with these people. And then CALO would actually contact them. It would look up your contact book. If there was a phone number, it would call them—if it was an email, send them an email or whatever—and coordinate and find the time. If it had access to your calendar, then it would use that. It would actually find the time, block the calendars, invite everyone. And then on the day and time when the meeting was going to happen, all you had to do is walk into the conference room and it would actually dial everybody out.
When I describe that, even today, we don't have a system that does that that easily for us even right now. So yeah, I think it’s prescient only because it's a problem we still have. The original Dejima people wanted to program their VCR. I don't know if you remember back then, but that was a thing—”I wish there was an AI system that could program my VCR.” So that was the original use case that we worked on was how do you program your VCR using AI. You still don't quite have that. Hopefully with generative AI systems and interfaces, we will finally get it, but it's been like 20, 30 years now.
Well, and you said that's still as difficult a problem today as it was then. Setting up meetings, answering emails, writing reports—why is that still so difficult to pull off today?
I mean, we have the components. I can tell you, we set that up as part of the CALO project. So it was actually working. The question of why there is no product that actually does that comes down to the viability of a business case for it more so than the actual technology. I think it is possible to do it. How often do you use Siri? We talk about Siri. I personally don't use it that often.
Why don't I? I had this traumatic experience, the first time I actually set up the precursor to Siri. There was this microphone set up in front of a TV set, a DVD player, and a satellite with 500 channels. It turned on the lights and had all this functionality. One of our advisors, the former chairman of Borland, was there. I just sat him down and gave him the mic, and said, "You can say anything, go ahead." And he just looked at the mic and said nothing. It was an awkward minute or two of him not saying anything. I asked, "Why don't you just say something?" He said, "I'm thinking." And he finally turned to me and said, "Well, I don't typically talk to my TV set, so I don't know what to say."
So there's that side of it as well. Even if we put that functionality in a system, will you talk to it? Would you anthropomorphize your system to the point where you would trust it to do what it can do, and talk to it in natural language? How often do we talk to our Alexa system in the corner? It's just a cylinder sitting in the corner of the house, and I'm sure it does tons of things, but do we really talk to it that often? No, it's just a cylinder. We're not used to talking to a cylinder.
Well then, I wonder, where do you think AI could have the most impact on how we work and how we get stuff done today? I mean, is it organization? Is it search? Is it collaboration? Is it something else? Where is your mind at right now?
Today, most of at least the folks that we see are scratching the surface of applications and use cases. It's mainly around, "Oh, I want a ChatGPT for X," or "I want to be able to do a document search on my proprietary document repository," or “I want to make my developers more productive."
But I think we're going to move to using this agent-based concept and start augmenting and improving our business workflows using these agent systems—which means that we're actually using them for the reasoning, for their ability to make decisions kind of out in the wild and make calls to the tools that we provide to them, like the API that we might have, or what have you.
So, I think we will move to that point. It's a leap of faith, though. If you have this technology that potentially is a knowledge worker for you, you can do all sorts of things. But how do you constrain them? How do you make sure that they operate in a responsible way? Who is responsible when they screw up? There are a lot of those types of questions that we need to answer. But on the other hand, they're so useful and they can do so much that I think they will start infiltrating our organizations and and improving all manner of decision making and business workflows.
At Cognizant, you lead this R&D team that helps bring advanced AI solutions to businesses. What are some of those workflows, or maybe some of the biggest challenges, that you've been helping companies address in your role so far?
We start with the KPI. We're like, tell us the KPI you care about, and let's work back from there. Because we want our AI to be aligned with you as far as what it's maximizing and minimizing when it comes to the KPI. I'll give you an example. Let's say we're helping a retailer make decisions with respect to its supply chain. The decisions could be things like which carrier to hire for a particular delivery, whether to change the delivery route, how many runs they should take, etc. But primary to all of this is the KPI. What are we trying to solve for? We want the shipments to be timely, we want to minimize our costs, and we want to maximize revenue or some other top-line KPI that we care about.
So, now we have the whole scoping of our use case. Here are the actions you can take, here are your degrees of freedom, here are the outcomes that you're trying to optimize for, and here's the information you have—like, what carrier am I running right now, or what am I actually moving, and stuff like that.
So then the next thing we do is we actually line up the generative AI-based agents. We give them the tools, which is, “Oh, I have this predictor model that can predict this KPI or that KPI. I have this optimization that I can do that gives me some sense of what actions to take.” And then the good news is these agents can talk to each other in natural language. So they're very, very robust. As things change, attributes change, new data comes in, they can account for that. And they can talk to us in natural language as well. So they can give us their best estimation as to what to do for a specific route. And they can also answer questions about it like, “What if this carrier is not available?” Or, “You know what, I didn't have a very good experience with this other carrier. Do you have any other suggestions?”
So that's just one use case, but you can use it for procurement, for manufacturing, for your support desk, you name it. So for some of these, you can actually defer to the generative AI's world model as well, which is something you would do with a knowledge worker.
So in the examples that you just gave, we're talking about KPIs, we're talking about the business objectives that are possible. I'm wondering, on the level of an individual knowledge worker, what does success look like for a deployment for employees? Is it time saved? Is it reducing the amount of toil that you spend on a particular task? Is it increasing productivity? What does success look like in that context?
So, a human knowledge worker is faced with decisions they make all the time. A lot of those decisions are informed by their expertise and their experience, but that could cut both ways. So they might actually miss certain aspects of a nuanced decision point. And so actually having a generative AI-based knowledge worker on their side that can help them and expand their horizon of what they look at before they make a decision, and allow them to consider alternatives, is actually helpful. It's useful. So it's not just the efficiency, it's the quality of the decisions that you're impacting as well.
And I'm curious as well, if an agent like this or a system like this can save us time, can make us more productive, what does that free us up to do more of instead?
A great question. I can tell you, I don't know the answer to that, but every time a technology as disruptive and general-purpose as this has come around that has made us more productive, it has made us busier. I guess in some ways we would want to look forward to a world in which we have fewer things to do. But I don't think that's going to happen. I actually think that we, by virtue of being more productive, are going to have more things to do.
We're always going to be a step ahead of this. I think generative AI is like a calculator: all of us should be using it and all of us will be using it, and all of us will be thinking of ways to use it to make the world a better place—hopefully, most of us. I think that's our role. Staying ahead and mastering this technology for the good of humanity is what we're always going to be doing.
Where have AI agents had the biggest impact on your day-to-day life lately?
I use AI agents for a lot of things in my personal life, as well as work life. It's my best coding buddy. I use them to write code. I use them to make decisions and choices when it comes to how I'm going to approach critical meetings and workshops, especially if there's a client involved and I really want to know what is the best approach to talking to these folks.
It's amazing how powerful these things are. Like any other tool, after you play around with it a little bit, you get a knack for how to use it and where it falls short. When you start using it, you start using it more and more and for more things. Now it's kind of a little bit of a cheat. When you run into a problem that you have to solve, your first inclination is, can generative AI help me here or not? So yeah, definitely.
What is something that you wish AI—generative AI, an LLM, whatever it may be—could do or a problem it could solve that it can't yet?
That's a tough one. Because these are general systems, there's a lot they can do, but it's on a continuum. There are certain things they can't do very well. The wish is more about them getting better at specific tasks. Can they get better at math? Can they get better at writing code? Can they get so good, as far as their context window is concerned, that I can give them an entire book and have them read it and then give me some tips on it or something?
There are some fundamental weaknesses that generative AI systems have, and that's a challenge for us working in AI to overcome. One of them is the fact that they're not embodied, they don't learn as they go. They're very generalist, which has its advantages, but every time intelligence has evolved in the natural world, it's been a learning system that adapts and learns in its environment. You would expect that from a machine learning system, but fundamentally, at its core, a generative AI model is a deep learning neural network. It's very difficult to have it intrinsically be learning as it goes. You have to play tricks to make it mimic that. So that's one area I think is important for us in the AI world to try to solve. I really do think that we're a few major breakthroughs away from being able to crack that nut.
Well, and we've also been talking a lot about natural language—things like talking and writing. But I also wonder whether there are other forms of interaction that are going to be increasingly important for us to consider as well, especially when it comes to people who maybe have different abilities, right? Maybe those who are visually impaired or deaf, where the things that we're talking about as “natural” don't come as naturally to them.
The architecture at the core of large language models is a transformer architecture. As long as you can pose anything as a string of tokens, these architectures are actually quite good, surprisingly good, even on visual-related tasks. The latest models that are coming out do have some multimodality. For example, Gemini is an inherently multimodal system. So if video is considered a string of tokens and you can see it as that, then a video feed could be part of the input to a generative AI system. So you can talk, gesture, use sign language maybe, and the system would still understand that.
Now, the main issue we have right now is that to enable that we need very, very large models. The larger they are, the slower they are, and the more expensive they are to operate and train. On the other hand, like any other technology, initially you get the big slow version, and then we as humans keep optimizing and make them faster and smaller. That is definitely the trajectory we're on. Hopefully, in the next few years, we will get to a point where those other modalities are viable.
So throughout this conversation, I've slipped into using the word agent because we've been talking about agents a lot, but I know that people also use other terms to describe the AI tools that are available today. You hear co-pilot, you hear assistant, you hear helper, even companion. What feels like the right frame to you?
I like the word agent, and the reason for that is because it actually forces you to think of this system as a knowledge worker versus a knowledge retrieval system. I think that distinction is very important. You don't want to rely on its learning corpus to come back to you and do stuff. It's pre-trained, that learning is outdated very quickly, and who knows what kind of biases or whatever else is in its world model. You really want to give it some tools, like you would to an agent, and then give it a task and have it go do that task for you. So I do like the word agent.
Co-pilot is good if we want to give people the comfort that it's always going to be side-by-side with a human—but not always. Why? There are a lot of tasks already that we delegate to technology to do on our behalf. Why should every use of AI be a sort of co-pilot usage?
Well, and you've used the phrase embody a couple of times as well—this idea that these aren't systems that are fully embodied yet. What will it take to get there? What needs to happen before we can have a system that does more of what you're describing? That ability to learn, that doesn't just rely on a pre-trained corpus of information?
We need some major breakthroughs to be able to do that. Right now, we use back propagation, which requires a lot of data. And you have to go through that data over and over again to nudge these neurons to do what you expect them to do, so the training is necessarily offline. And then post-training, we do some fine-tuning. You are really not changing the world model as much as just tuning it to prefer one over the other. And none of that is very satisfactory.
There are approaches that are much more efficient. Evolutionary computation is one. There are symbolic approaches that, within the world of AI, people have worked on. And I think if we look at generative AI as a proof of existence—in other words, if you can scale a system the way we have, and task it with a problem like language modeling, the way we have—there will be emergent behavior that ranges from reasoning and some math to poetry to language to all that kind of stuff. So, that proves that there is at least one path to get there.
So maybe we can use elements of that, but use a completely different approach that would be more explainable, would be, actually, conducive to online learning and to correcting and modifying the world model of these systems as they go. That’s when that, paired with much more efficient systems, would get us to an embodied version. There is a proof of existence of that as well, which is the human brain. So we know that is also possible. It does start more or less from scratch and learns and absorbs. And it can change its world model. With one instance—you show it one example, one counter example—it modifies its world model. It's very plastic in that sense. So, why not expect that plasticity in our AI systems?
We're talking about the future right now in a sense, and I'm wondering, what are you looking forward to in the future, both professionally, but also with some of the ongoing development in AI more generally?
In the shorter term, I'm looking for more efficient, more powerful models, for sure. I’m also looking to see people adopting this beyond the obvious. Like most of us, our first experience with generative AI was ChatGPT. It's a chat interface, and it can do certain things that have to do with language or writing code. So most of the use cases we see out there are kind of offshoots of that. But as I mentioned, there's so much more that can be done, so I'm really looking to that flourishing of use cases and the pervasive use of these systems.
In the longer term, I want to see breakthroughs. In fact, in some ways it's not a good thing that we're myopically focused on one architecture, one way of training a model, and one kind of use for these generative systems. The field of AI is much, much, much wider than that. And everyone seems to be focused on this one path that has resulted in some fascinating breakthroughs, but it's just one. And in the future, I do think that we need to start exploring. We need to be more creative, so that we can overcome some of the challenges of this particular approach.
I think that's a good place to leave it. Babak, thank you so much for joining us today. It's been really nice having you here.
It's been awesome. Thank you. And really, really good questions. You kept me on my toes.
Thank you.
~ ~ ~
Another thing I remember from when I was younger was the first time I tried to talk to a phone. Not on the phone, but literally, to it. It was an old Nokia, like T9 era, that had some very basic voice recognition on board. It didn’t matter how hard I tried, I could never get it to work. But we’ve come a long way since then. Our assistants are pretty good now! Whether I’m asking my car to change the music, or talking to ChatGPT, it feels like we’ve got the recognition part down.
But as Babak says, where things get real interesting is when we go beyond the basic commands, the question and answers, and throw reasoning and decision making into the mix. Already, we’re starting to see AI-powered tools that anticipate what you need and when you need it—without you even having to ask. The kinds of tools that won’t just do what we tell them, but actually understand our intent… whether you’re trying to prep for your next big meeting—or, just want to watch tennis at the bar.
Working Smarter is brought to you by Dropbox. We make AI-powered tools that help knowledge workers get things done, no matter how or where they work.
You can listen to more episodes on Apple Podcasts, YouTube Music, Spotify, or wherever you get your podcasts. And you can also find more interviews on our website, workingsmarter.ai
This show would not be possible without the talented team at Cosmic Standard: Our producers Samiah Adams and Aja Simpson, our technical director Jacob Winik, and our executive producer Eliza Smith.
At Dropbox, special thanks to Benjy Baptiste for production assistance and our illustrators Fanny Luor and Justin Tran.
Our theme song was created by Doug Stuart.
And I’m your host, Matthew Braga. Thanks for listening.
~ ~ ~
This transcript has been lightly edited for clarity.