Working Smarter
Episode 5: Natalie Kupperman on why we still need humans in the loop
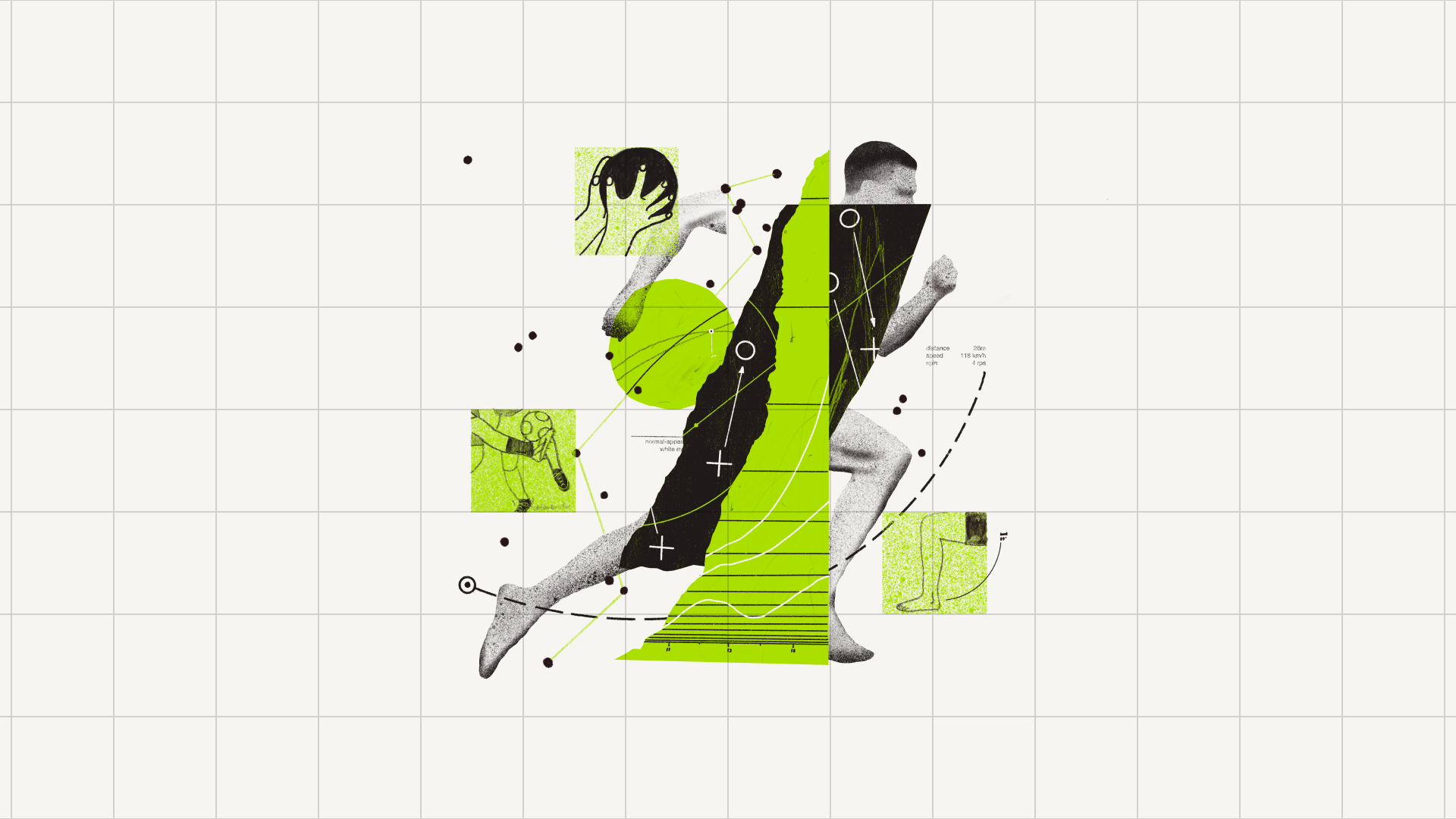
For our fifth episode of Working Smarter we’re talking to sports scientist Natalie Kupperman. She’s an assistant professor of data science at the University of Virginia where she studies the use of biometrics, wearables, and AI-powered tools for athlete monitoring in sport.
Whether it’s a machine learning algorithm trained on video footage from practice, a force plate sensor in the weight room floor—or some combination of the two—Kupperman is interested in how new technology can help reduce the risk of injury and optimize athletic performance for professional athletes and amateurs alike.
Hear Kupperman discuss a day in the life of a sports scientist, the importance of getting all of your data into one place, and why there will always be some injuries we can’t prevent.
Show notes:
- To learn more about Kupperman and her work, you can visit her website or her University of Virginia faculty page
- Find Kupperman's research on Google Scholar
~ ~ ~
Working Smarter is a new podcast from Dropbox about how AI is changing the way we work and get stuff done.
You can listen to more episodes of Working Smarter on Apple Podcasts, Spotify, YouTube Music, Amazon Music, or wherever you get your podcasts. To read more stories and past interviews, visit workingsmarter.ai
This show would not be possible without the talented team at Cosmic Standard, namely: our producers Samiah Adams and Aja Simpson, technical director Jacob Winik, and executive producer Eliza Smith. Special thanks to Benjy Baptiste for production assistance, our marketing and PR consultant Meggan Ellingboe, and our illustrators, Fanny Luor and Justin Tran. Our theme song was created by Doug Stuart. Working Smarter is hosted by Matthew Braga.
Thanks for listening!
Full episode transcript
For about a decade now, whenever I’ve gone running, I’ve worn a smartwatch on my wrist. Now I’m obviously not a professional athlete, and so the stuff I’m tracking is relatively tame. Distance, pace, heart rate—nothing fancy. But after three marathons—and I’m about to run my fourth—I honestly don't know how I would train without it.
Whether it’s hills, speed work, intervals, long runs, my watch makes it easy to follow a plan. And the trends and patterns I can see in the data don’t just help me train—they keep me healthy, and tell me when I need to rest. Because I don’t just want to run faster, as nice as that would be, but run smarter, and more sustainably, too.
Marathons, if you’ll pardon the pun… are a long game.
Now, I’m only scratching the surface here. In fact, there is a whole world of data science in sport dedicated to getting the best performance out of athletes and their teams. Wearables like mine are just the start. There’s smart rings, cameras, countless apps and sensors.
And like a lot of jobs, this work increasingly involves AI.
I’m your host Matthew Braga, and on today’s episode of Working Smarter, I’ll be talking to Natalie Kupperman. She’s an assistant professor of data science at the University of Virginia where she studies the use of biometrics, wearables, and other new tools for athlete monitoring in sport.
Whether it’s a machine learning algorithm trained on video footage, a force plate sensor in the weight room floor—or some combination of the two—Natalie is interested in how technology can help reduce the risk of injury and optimize athletic performance.
Now, what does that look like in practice, so to speak? That’s coming up next on this episode of Working Smarter.
~ ~ ~
Natalie, thank you so much for joining us today.
Thank you for having me. I'm excited to be here.
Maybe to start off you can tell us who you are and what you do.
Yeah, my name is Natalie Kupperman. I'm an assistant professor of data science at the University of Virginia. I'm clinically trained as an athletic trainer. And for those people who don't know what an athletic trainer is, it's the person you see on the sidelines of sports who runs out when someone's injured and provides medical care. They also provide medical care for non-emergent situations. But that's where my background is, and it's very clinical. I got into data science through that.
I was working at Northwestern University when they started using these Catapult sensors with the football team. I had a master’s from UVA and people looked at me and said, "Hey, you like to do stats. Why don't you take all this data? They say it's going to help us prevent injuries. Let's see what it all means." And I quickly realized that it was a lot of data that I could not run in Excel or in any of the older stats programs. So I ventured into data science and eventually got a data science certificate, and then went back for my PhD. And now I'm here still trying to figure out if we can prevent injury with wearables.
Okay, well, we’re going to get into that, but first I have to ask, what is a Catapult sensor?
I figured we'd get into this. So there's lots of different sensors on the market. Catapult was one of the first ones out. Around 2015 is when it started to get popular here in the U.S. And it's a sensor that's gotten smaller over time. For the longest time, the athletes would wear this in between their shoulder blades in a harness that resembles kind of a sports bra. And now you can wear them either still in between the shoulder blades or at the hip. It is a triaxial accelerometer, gyroscope, and magnetometer—so it's tracking accelerations and also the orientation of the athlete. It gets raw data, but then also does a bunch of processing where we get different variables that give us an indication of how much workload an athlete is doing on field or on court.
So a little bit more intense than what you or I might wear to go out on a run.
Yes, yes. And actually, a lot of the watches are doing similar things now because the technology has gotten better. But it would tell you, like, if you were out for a run, not only how far you ran, but how many times did you accelerate or decelerate during that run. Or how hard were your footfalls, maybe.
Got it. When you were first asked to look at these devices and the data that they generated, do you remember what was going through your mind?
This is so cool. [Laughs.]
[Laughs.] How so?
Athlete tracking has been going on since, you know, the Greeks started the Olympics. When we measure “How much do people lift?”—that's athlete monitoring and athlete tracking in the simplest form. And as an athletic trainer, I’m thinking, wow. We often think about overuse injuries, and we're often trying to figure out, like, how do we quantify what's happening to the body day-in and day-out on the field? We can use time. We can know how long somebody's out there. Or maybe we can calculate distance. That's not as easy in team dynamic sports. But I'm thinking, wow, now we're getting this rich information data set on individual athletes every single day. And when we have all this data, hopefully we can start to find some insights into how we could prevent, specifically, overuse injuries.
Right. And I know that when you talk about data, and data and sports in particular, it's hard to avoid the reference to Moneyball—which, great film. But that's obviously a very narrow and specific thing. So I'm wondering, how do you define that larger branch of sports analytics and all the things that it can encompass?
I typically divide it into two sides. So we have sports analytics, which I think is much more of the Moneyball front office-type analysis, and even the technical, tactical pieces of game strategy, gameplay analysis. The other side, I would call that more sports science. Now we're looking at human performance, injuries, wellness, those types of things. And what's fun about that is that, not only can we do that in athletes—that trickles down to everyday athletes, the people who go out for their jog every morning, or who are training for marathons. And so I really like working in that sports science space because it does have a lot of applications beyond college athletics or professional sports.
So broadly speaking, when we talk about AI and AI-powered tools, how are those being used in sport today?
We've had a lot of products for a while that have a machine learning component built in. And that machine learning component might be deep learning or neural nets that have an AI component to it. I would say, though, that the big frontier in sports where AI is going to play a really big role is in video and video tracking. Listeners might be familiar with tennis. The U.S. Open was one of the first to implement what's called Hawkeye. It's a company that has a video system. And so when a ball is on the line, often you'll see a replay that's computerized. It kind of shows where the ball landed on that line for viewers, and then they also use it to help make calls.
Hawkeye is really good, historically, at tracking the ball. Similarly in baseball, it could track a whole player pretty well, and gave us a lot of insights on how players are on the field compared to opponents. Let’s say the baseball athlete got a double. Now we can know, how did they get that double? Like, where was the team that was pitching on the field when that was happening? And so we're getting a whole view for game strategy, which can really start to change the game and how coaches approach the game.
And would you say it's primarily video at this point, when we talk about sort of ML applications specifically? Or are there other things that come to mind?
Video I'd say is the next frontier. A lot of teams don't have video. The video is largely at the professional levels, because it's very expensive to run, to maintain, to analyze, to store all of that. At the college level, we see a lot of wearable technologies. We mentioned Catapult. The Oura ring is commonly used in athletics for athletes to track sleep, and now they track daytime stress and all of that. In the weight room we often see a lot of technology in the weight racks. That could be both camera or sensor-based. And all of that information feeds somewhere, depending on how their data infrastructure is set up. And what we're seeing, too, is a lot of companies that have been created to help pipeline that data and analyze that data using machine learning.
A second ago, you were talking about that evolution from video-based systems being able to detect maybe a ball or a piece of equipment to—now they have the capability of not just detecting maybe one person, but multiple people on a team, the entire field of play.
And the big thing for that is now it's working hard to be able to detect, like, individual joints and specific biomechanical angles of joints.
Well, and that’s a really good segue because I think what I was wondering is, you know, what are the implications when you can go from being able to detect a ball, a piece of equipment to the entire field? And not just the entire field of play, but how people are moving on the field? What are the implications of that for health and athlete fitness?
We could first talk about it in terms of game strategy and the World Cup.
Uh-huh.
So the World Cup, we saw FIFA implemented offsides being looked at using the video system that they had. And that was really tracking, like, if your knee was over the line by a centimeter, you were offside. Where before we had to use a human to do that, right? We had to use a ref. So what was the implication of that? We'll never know, because that's the fun thing about sports. We can't go back and figure out how the games would have changed. But did that give teams that tended to play by the rules or not outmaneuver the refs an advantage? Versus, there are teams that were really good at being offside when the ref's not looking—and now they're getting called out. And does that hurt them, you know, in their progression in the World Cup? There are blogs out there that say yes, yes it did.
And so now we're not talking about a win. Like, okay, every athlete wants to win. But now we're talking about major money, right? If you get out in the first round of the World Cup versus winning the World Cup, that's a big difference in money for your team—possibly for you, depending on their bonus structures.
Totally.
All because a computer is now deciding offsides. And the refs had to confirm with the technology, it wasn't one sided. There was still a human in the loop there. But we have to wonder. And then that's going to change how teams now go forward with strategy. Don't be offside, ever.
And then what about in terms of health and fitness? Like, when you're able to then get that sort of granular data on how athletes are—not just where they are on the field, but how they're moving? What are the implications of that?
I think first it will help us determine how some injuries occur. We can look at ACL injuries. So it's a ligament in the knee. Unfortunately it's a pretty commonly injured ligament in soccer and lacrosse, and more in women than in men. And it usually has to be surgically repaired depending on what you plan to do for the rest of your life, or what kind of sports participation you want to get back to. And often, specifically in women, ACL injuries are non-contact. So there's nobody else around. It's not as though a collision happened and your knee got twisted underneath you. You're running, and you pivot, and your knee gives out.
We know this, and we can go back and look at, you know, like the practice film. But we don't have a great angle on that player. But if we have the newer cameras and all of the software that comes with them, now can we look at that knee's exact biomechanical angle? We'll never know exact ground forces, because we don't have force plates on the field. But through great mathematics, can we figure out what that ground force reaction might be? And then can we start to understand why certain people have these non-contact ACL injuries based on their specific biomechanics.
Interesting. You also mentioned force plates a second ago. Are those in use already?
Yes. I would say force plates are very common in the weight rooms in college and professional sports. The degree to which they're used varies depending on where you're at. And that's very much who's running the weight room or the performance center and their aptitude for technology.
So within a typical sports team or organization, what does a day-in-the-life look like for a sports scientist?
I can speak to kind of how my day-to-day would roll. Here at the University of Virginia, I do some sports science, data science work with the men's basketball team. Typically guys come in in the morning for their lifts. A couple of times a week the athletes do wellness surveys. We check in, ask them how they're feeling on different dimensions. After they finish that, they're going to go warm up. Then we're going to move on to things like the force plates, doing something called a counter-movement jump. That data goes up to the cloud and then we pull it back down and we get a nice table that says: the different key metrics that we look at for the counter-movement jump—have they increased, decreased, or stayed the same for that athlete compared to some previous time frame for them?
That gives us an indication of something we call neuromuscular fatigue. So, is this athlete feeling really good, ready to roll, going to be able to do a lot of physical movement today? Or do we need to pull back and give them more of a rest? Because for a sports scientist, your goal is to make them as prepared as possible to play their sport. We always take out things in the weight room before we take out anything on the court. So I would help collect that data. The strength coach is the one who ultimately decides what exercises go and stay.
Also in here, we've looked at all their Oura data—so we know how everyone's been sleeping, resting heart rates, all of that. And then they lift their weights. They maybe do some recovery modalities. While they're lifting weights, they're also being tracked with cameras and they're tracking, you know, how many sets and reps they're doing. They usually leave for a while, then they're going to come back for practice.
Again, they come, they warm up, and they put on their Catapults and go off to practice. And then at practice, the sport scientist is usually live tracking the Catapult data or whatever wearable data there is. If the team's using camera data, you might be live tracking some of the camera data, doing things like periodizing it—like, “During this time frame, we were warming up. During this time frame, we were doing X drill"—just to set up your data infrastructure for later on when you're doing analysis.
And then practice ends, and you download it all, and you start looking at your analysis for the next day. And the time in between the weight room and practice, you might be having meetings with coaches about, what's this guy looking like today? What's this girl looking like today? As a team, are people feeling fatigued? When's our next game? What kind of modifications can we make to practice to make sure that they're prepared for whatever the next competition or next milestone they need to meet?
What are some of the most common pain points or struggles that you or sports scientists more generally encounter in these kind of scenarios? Like, is it information overload? Is it struggling to make sense of everything that you have access to?
At all levels, this is an issue. I've heard this at professional levels and at collegiate levels. The issues are data infrastructure, just like you said. There is so much data, and getting it all into one place where it's easily queried and then usable—and usable meaning dashboards for the people who want a lot of data, and they want it interactive. So dashboard building is not the hard part, but getting the dashboard set up exactly how somebody wants it and will hopefully use it is important. A big challenge is the data infrastructure.
The next one is that we can't control what happens outside of the performance center or outside of the court. A lot of the way athletes are feeling and if they're fatigued comes from stresses they're encountering in their lives outside of sport. And, you know, I think trying to figure out ways to quantify that so we can get a gauge—like, okay, so when athlete A isn't sleeping well, these are modalities that help them and help their autonomic nervous system recover. Most people will say the best way to fix sleep is to figure out why you're not sleeping. But sometimes that's really hard to get at. Sleep is going to affect your athletic performance. And so that's one of the most frustrating pieces of being the sports scientist, the data scientist, the coach—frustrating for everybody—is you want to control all the little things, but humans are the most probabilistic thing to study.
Well, with some of the data infrastructure issues that you were talking about—with making sense of the data, with corralling the data—how have AI-powered tools helped in that regard?
All the raw data—so the raw sensor data or the raw camera footage—runs through algorithms. And we get nice outputs with like clearly defined variables. If you're someone who is not as tech savvy, you don't want to get into the raw data. You just want to know: how many times did this athlete accelerate? How many times did they jump? That's all going to be output for you in a nice format — typically a CSV or JSON if you're going to use API piping. And then you can look at that and it'll give you pretty good idea of what's going on.
The issue there is you don't know how it got to defining that that was a jump. And so you have to weigh which one you want more. Are you going to trust the company, that the output that they're giving you is accurate? Or are you going to hire people to dive into the raw data and define it yourselves?
You have these systems now that can automatically identify players, track players, track their movements, and I imagine that's work that you, or people like you, would have had to do in the past manually. Now that you don't have to do that kind of work, what does that free sports scientists up to do more of instead?
So before, that really wouldn't have been a sports scientist's job. I'm trying to think who—I suppose maybe an intern?—would have watched film and tracked something. I remember back when, I guess even still now somewhat, if you wanted to know how many jumps somebody got, like a volleyball player, somebody—usually the grad student or the intern—sat and watched film and hand counted. You know, this athlete jumped this many times. And now what are they doing? They are figuring out how to pipe all that data into one database and make it easily queryable for somebody to analyze.
I feel like I've lived that. I feel like I'm talking about an evolution that I’ve lived. I never had a research project where I had to count jumps, but in that space, when I was getting my master’s, we were working with MRI data and muscle segmentation. Athletes would go get these MRIs to look at muscle volume, and we would have to go slice-by-slice through the MRI and trace each muscle in the leg. You might think they're axial slices, so you're going all the way up, and you're just like outlining the hamstring muscles, dot-by-dot. And I did this for hours and hours and hours for my master’s thesis. And now that technology is a company, and AI does it. They get the scans and it runs through and all the muscles are segmented.
And that's actually part of the reason why I ask. Because when you hear about the benefits of AI, you often hear about, you know, we have tools now that can make some of the rote work, the admin work, the repetitive work—we can automate that stuff. And we can take that away and leave you time to do more of, I don't know, another thing that you find more valuable or important in your job. And I'm wondering if there are examples of that you've seen so far for your field.
The easiest things to automate are analysis. The machine learning is fairly easy to automate for the most part. And that's everyone's favorite thing to do. When I talk to students and what they want to do, they want to do machine learning analysis. And I'm like, "That is automated now. We don't do that anymore"—unless you're going to create some kind of like novel approach. So we can put the data in now and we have AI methods—or machine learning methods, really—that can run through every different scenario for you and give you the most optimal output for whatever your outcome is. And then we can also go back and look at those and understand—like, we might not have wanted it to hyper-tune specifically how it did, but we can pick out the model that we want based on our other assumptions. But instead of someone having to hand code all these parameters, a lot of that is just packages now in Python and R. And then there's companies that also have that.
But what can't be automated is data cleaning. Some of it can be, but we still need a human to go in and look at all of that. Sensors are finicky and we can get a lot of aberrant data, and if it slips through it can really mess up our analysis. And so we have large chunks of it automated. But I would say that's the area where there isn't perfect automation, and it still takes a lot of human capital, which is usually everyone's least favorite part of the whole data pipeline.
This is a bigger picture question, but why does this matter for athletes, teams, and sports organizations? Why is there such an interest in using AI and technology generally for the sake of athlete health and fitness?
I think that answer varies by the user. So if we're looking at the team level, they obviously want their athletes to be on the court. We know from NBA case studies that when big players aren't on the court, fans get angry and they want to see their favorite athletes on the court. And teams also want healthy athletes, no one wants an injured athlete. So they're hoping that by tracking athletes and making athletes that are optimally healthy and less likely to become injured, that more athletes can be on the court and we're going to have less people on the injury roster.
On the athlete side, I think it varies athlete to athlete, sport to sport, league to league, on how much they engage with technology. Runners, like in college and professional runners, are typically people who are very engaged with their data. And I think they want to have long, successful careers. If you're a professional runner, you want to run for as many years as you can. This type of data can help you really get down to the nuances of your body. When we get to an elite level of sports, we're really splitting hairs on incremental improvements in performance. And the way we can get that .025% in performance—which could put you in the top three versus the top 20, which is going to the Olympics versus not going to the Olympics—might come down to these very intricate things about your human physiology.
Being able to collect data on you—if we keep with the running example, every step you take—throughout your entire recovery modality sequence, that's huge. And that can really take you from being a mediocre runner to a runner who is highly successful for many years. Especially if you have a team of people around you who both understand the data and also understand the art of coaching. I personally don't believe we'll ever get to a point where we're just running on numbers. There's always going to be that intuition, right? People know their bodies and coaches know athletes, so I think there'll always still be that relationship.
You sort of alluded to this a second ago, but I know at Dropbox we often talk about AI as this human-machine partnership. How important is the human element in this kind of work?
I think it's hugely important. Especially since we're working with humans. I think that, you know, the machines can give us a lot of great data. Hopefully it helps make our jobs faster so we can work on more futuristic, forward-thinking ideas, versus spinning in the same spot. And I hope it never comes without human input. I never hope that games are run based on what this algorithm says the next play should be. Because there's always going to be that human intuition.
We talk about that when athletes walk into the performance center. When you're a coach who's worked that athlete for the last three years and they come in, I think everyone can understand that sometimes you just get a vibe off of them. You're like, something's not right. Even if you've answered your wellness questionnaire perfectly, you jumped beautifully—like, I could just tell that something isn't quite right. And that's where the technology is really amazing at being a conversation starter. Because maybe we did notice a strategy change on their counter-movement jump, but we also need that human to be there to be like, “Okay, what's, what's really going on?” Because often what's really going on is happening outside of sport.
From a fan perspective, we all love watching sports because it's so humanistic, right? There's so much emotion in it, we don't know what's going to happen. There's like the underdog stories, you know, all of this. And if the human components aren't there, like what fun is sports? It's like watching computers play chess—which is a cool academic exercise, but doesn't quite have the same level of emotion behind it.
A little different.
A little different.
When you talk to teams and and organizations, what's the thing you hear most frequently from them that they're hoping to solve with AI?
Getting their data in one place. I'm telling you, it's a bigger problem than people realize. But what we talk about a lot, specifically when we're talking about game strategy and tactics, is getting a sense of the whole court. Right now we mostly either know where the ball is, or where a person is. But when we are thinking of team dynamics, we need to know where everybody is, and where everybody on the other team is. And that technology exists. It's still very much in the professional sports, and yet to trickle down to a lot of like college level. But even when professional teams have that data, I think it's still kind of a data deluge. And so to be able to sift through that and figure out what's important here is something they're hoping AI can help them solve.
What about in terms of injuries? Do you hear a lot of people coming to you and saying, “Hey, can we use this to predict or to prevent our athletes from getting injured now?”
Yes, I hear that that a lot. Usually when I walk in to talk to teams, their number one question is like, “I just don't want any injuries anymore.” That's never going to happen.
Why not?
Injuries are always going to come with sports. It's just part of the game. Maybe if we can get rid of overuse injuries, that would be awesome. But I'll never be able to stop someone from stepping on somebody else's hand and fracturing their hand. There's just always going to be those injuries that just happen because we put very large humans on courts and they run into each other. It’s the nature of the game.
But this is really challenging too when I talk to teams, because we have so many companies out there saying that their cameras can get biomechanical angles and we can prevent injuries and predict injuries. And I've yet to actually see that in the academic research. So I feel like some of the companies are a little cart-before-the-horse type of situation, where they're giving a lot of promises and we don't actually know how reliable those algorithms are for looking at things like joints or distance. That kind of thing is off the camera, and we still have a ton of work to do on the computer vision side for this.
So while that is an amazing pipe dream, and I would love to be able to track the athletes all the time on the court, and be able to get these really great models of injury risk mitigation, we're still a ways off from it. think our best hope right now is just to triangulate the camera, the wearables, and just using what we know about athletes. If you're feeling tired, when you're feeling fatigued, you're probably more likely to sustain some type of fatigue related injury. So yes, it's coming, and I'm very excited about it, but I think we're further off than people realize.
What else is on the horizon for the near future that you're excited about?
Specifically in athlete health, it would be—this concept has been around for a while now, but I think AI is actually helping us get there faster—this concept of a digital twin. We're collecting data every day on athletes, so we have a lot of data on one athlete. We can kind of construct a digital version of that athlete. And now we can do simulations where we simulate what the next practice might look like for this twin. How do we predict or think that their physiology will change based on these different options? And I think that is so cool. We can do it now, I just think AI will speed that up and make it—the important part here is making it more accessible for people who don't have a team of data scientists and engineers and sports scientists all working on this for 16 athletes.
I've heard a couple people now talk about how their particular industry had, like, a ChatGPT moment where ChatGPT came along and suddenly they were thinking about the implications of AI for the work they do. Is there an example of a big, recent advancement in AI and sport that had a similar kind of outsized impact on the way that people thought about athlete monitoring, or maybe just analysis more generally?
For athlete monitoring, now I'm thinking about, in the NFL, the next-gen stats. I remember those first few games when the speed would pop up over the player's head, and people were just like, "Whoa, like this is amazing.” And they were using RFID tags to do that. But I think that was a real moment that was like, "Wow, we can get really precise measurements in the actual environment in which they're playing.” Whereas before would have to do that in a lab. And I think from the health perspective, what we're trying to do now is actually figure out ways that we can look at that data and causally link it to injury—which isn't an overnight process, especially when there's a sampling error in the fact that not every athlete gets injured every day, or even every season. So we have a lot of data and not a lot of injuries. And so understanding those causal mechanisms is really challenging. It's hard to do that with AI and ML because we don't always know what's happening under the hood there.
Well, and this comes up a lot, I think, when we talk about AI, about how it's such a new technology. How important is it to be open and transparent about what kind of data is being collected and what's being used to train these models? Basically informing users, like, “Hey, here's what goes into giving you all of that analysis that you find so useful.”
I think athletes need to understand when they're being tracked, why they're being tracked, and how that data is being used to make decisions on them, specifically related to their career projections. And it's really challenging in athletics, because we can say—and with the teams I work with, we do the best we can to do a consenting process. But athletes can feel that they need to consent, because they're going to think that the coaches are going to know if I don't consent. Like in basketball, specifically, when the draft time comes up, teams call and say, “Hey, I need this data set on the athlete.” And it includes things like height, weight, body fat. I can't call your doctor and ask for your weight. So it's weird that teams are just like, “We’re just going to have you send us this information on this athlete.”
And then the athlete’s put in a real bind too, because they might not want it sent. But they feel like if they don't, then they're not in the mix. But if they do send it, they don't know how that data is being used for or against them. There's a lot of opaque processes and that will continue to play out, I think, over time. But if we could get some best practices in place, I think that would start moving things in the right direction.
Throughout this interview, it’s obvious that you’re really passionate about sports, about data analytics. But I’m wondering if you can tell me a little bit about why. Like, what excites you most about this work?
I love the emotions behind sport. I love being around sports. I love being around that team mentality. I love helping one person succeed or one team succeed in whatever succeeding means. I mean, that's why I'm also in academia. I love being around students and helping students achieve their dreams. It's easy to get swept up in it. And then I like the individual aspect of health, and I also feel like it's one of those areas that can hopefully be generalized to the recreational runner—you know, to me. I'm not an athlete, but I want to stay walking until I'm well into my 90s. How is my Oura ring going to help me? I think we can study it in elite athletics, and then there's a trickle down effect. And maybe there's a trickle up effect too. Yeah, I think that's why.
Natalie, thank you so much for joining us.
Thank you for having me.
~ ~ ~
Have you ever noticed how corporate America loves a sports analogy? They’re always talking about keeping their eyes on the ball. Or dropping the ball. Or getting the ball rolling—and that’s assuming they’re not curveballs, by the way.
There’s playing offense, and playing defense, or playing to win.
I think it’s because work, like a lot of sports, is a team effort. And a team is only as good as the people who are on it. No matter how good the equipment or gear, it’s still people who—uhhh, what’s another analogy? Hit the home runs?
When I think about my running, and how I train for my races—I’m not just doing whatever my watch says. I also have coaches. I run with a group. I work with people—my team—to figure out a plan. My watch just helps me execute. And that should also be the case with any AI-powered tool.
That said…I’m a bit intrigued by this digital twin that Natalie mentioned. Maybe AI Matthew can just run my next race for me? Just this once?
Working Smarter is brought to you by Dropbox. We make AI-powered tools that help knowledge workers get things done, no matter how or where they work.
You can listen to more episodes on Apple Podcasts, YouTube Music, Spotify, or wherever you get your podcasts. And you can also find more interviews on our website, workingsmarter.ai
This show would not be possible without the talented team at Cosmic Standard: Our producers Samiah Adams and Aja Simpson, our technical director Jacob Winik, and our executive producer Eliza Smith.
At Dropbox, special thanks to Benjy Baptiste for production assistance and our illustrators Fanny Luor and Justin Tran.
Our theme song was created by Doug Stuart.
And I’m your host, Matthew Braga. Thanks for listening.
~ ~ ~
This transcript has been lightly edited for clarity.