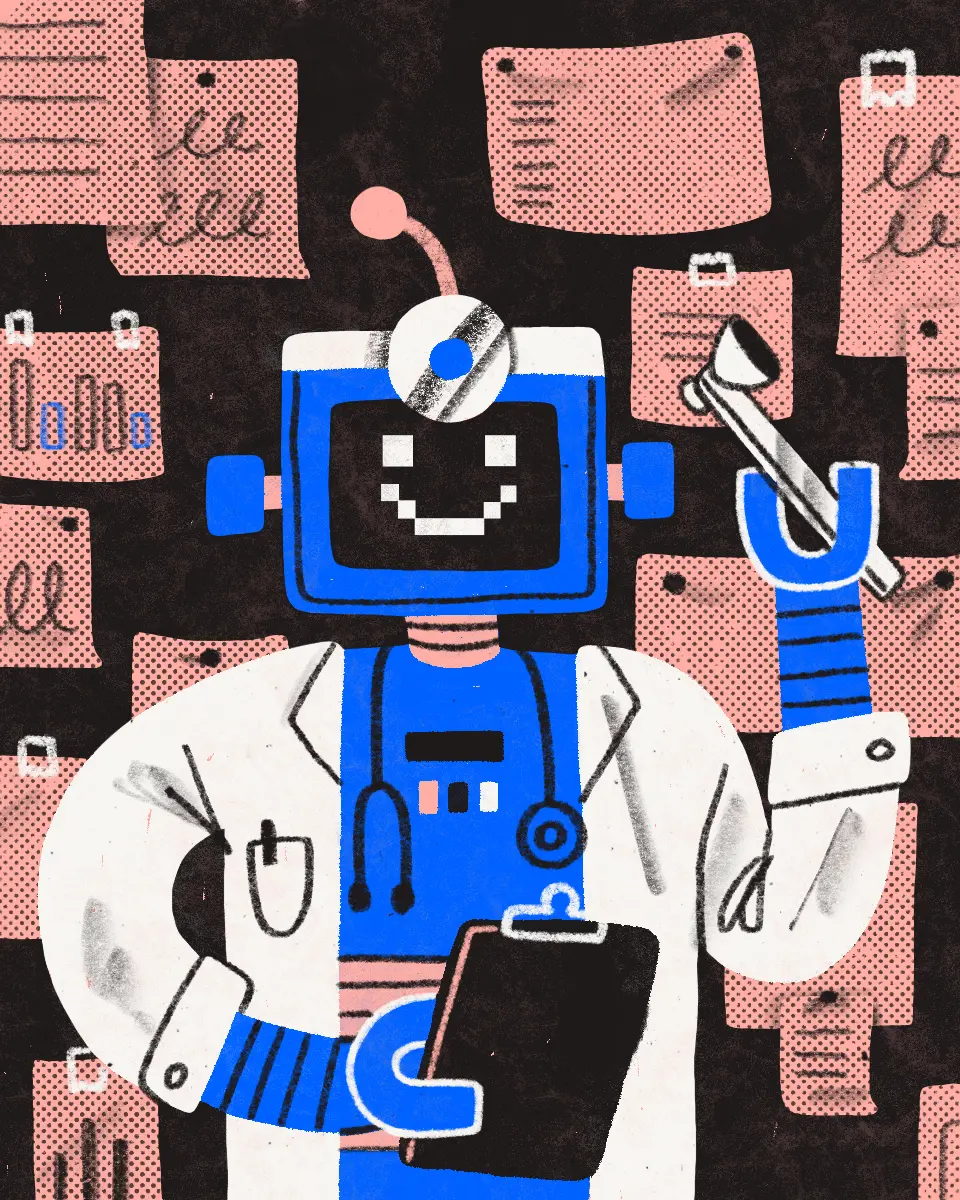
Meet the AI researcher helping doctors choose the right cancer therapy
Published on April 07, 2023
In our new Working Smarter series, we hear from AI experts about how they’re leveraging machine learning to solve interesting problems and dramatically change the way we work for the better.
While studying pre-med at Johns Hopkins, Rohit Bhattacharya lost a close friend to cancer. The loss inspired him to pursue a career in machine learning in the hopes of building tools that can help doctors determine which therapies are best suited for which patients.
Today, Bhattacharya, Assistant Professor of Computer Science at Williams College, is chasing that goal by training machines to understand causal inference—the cause and effect relationship between genetic data sets that have been far too large for humans to perceive. “The beautiful thing is that we all care about cause and effect,” he says. “It's just ingrained into us—we all want to understand what caused something else.”
When were you first introduced to the concept of artificial intelligence?
I think it was when I was playing video games as a kid. I realized there weren't little people inside of the TV playing against me. It was something else. That's when I realized you can write programs to perform certain tasks with human-like qualities.
How did you land at the intersection of medicine and machines?
Actually, I wanted to be a medical doctor. I started out as a pre-med student at Johns Hopkins. I was doing biomedical engineering. Then, in my second semester of college, I saw the Da Vinci robot at Hopkins and found it fascinating. It's a surgical robot that allows surgeons to do more precise surgery, remote surgery, and things like that. In some cases, they try to automate the surgery—certain tasks like suturing. That's when I realized I was a lot more interested in being the person building those kinds of tools rather than being a doctor myself. I thought, as a doctor, maybe you serve one person, but if you build a tool like that, you serve several more people.
“The beautiful thing is that we all care about cause and effect... We want to understand what caused something else.”
You’re training machines to understand causality. Could this improve a chatbot’s ability to tell fact from fiction?
Yeah, I definitely think so. Misinformation is getting harder to detect. I'll give this simple example of sensational headlines. As soon as we see a super sensational headline, then we're less likely to treat it as reliable news. Sometimes it's about tone, right? What does the language look like? Is it sensational in some sense? It's hard to put your finger on it, but when you see it, you're like, that’s not quite right. There's something off here. Being able to encode that kind of intuition into language models would be really helpful. [It’s about] trying to encode that sort of baseline knowledge, go through a list of check boxes before we choose to believe something, and mathematize what [humans] do when we’re looking for misinformation.
As AI progresses from deep learning to deep understanding, what applications are there in your field of research? What do you hope to see in the next five years?
In terms of understanding the mechanisms of cancer, you want to know whether certain genes have a cascade—maybe a tumor suppressor gene then leads to a cascade that results in other genes being underexpressed or overexpressed, which then can lead to the shrinkage of your tumor.
There has been a whole switch to causal representation learning where you're looking for different kinds of patterns of association. There's the kind of association where X causes Y. There’s the kind where Y causes X. There's also a different kind of association, where maybe there's a third gene unknown to us—we haven't even measured [yet]—that causes both of them. We've adopted this new kind of technology to learn different kinds of representations, where we explicitly have a way of expressing the difference between an association in one direction or the other, or spurious association.
How does understanding cause and effect make the treatment process less complicated?
Causal inference has typically been focused on treatments of placebo versus the actual treatment. You focus in on this binary treatment of yes/no: Did you receive the new kind of treatment or the old kind of treatment? The mutations in our genome are not binary in the sense that they could be under expressed, over expressed, different levels of expression.
The other thing that's a lot more complicated is you don't suffer from one mutation. Usually, you get a multitude of mutations across your genome. Any one of your 20,000 genes could be mutated. It's an enormous permutation of how many kinds of mutations you could have, which genes have been mutated. How do we even think of that as being one version of the treatment versus another?
We'll never observe all permutations of mutations. It's physically impossible. So maybe we start to think about mutations and networks of genes rather than mutations of individual genes. That leads to this sort of thinking, where we live in a world where data is dependent. Mutations in one gene can affect others. In the same way, we live in social networks, where if I get the vaccine, that protects not just me but also others.
“Being able to encode intuition into language models would be really helpful.”
For a long time, we’ve sort of ignored this dependence in machine learning and statistics. We’ve pretended all data is independent, identically distributed, and have analyzed it as such. But we're going to have to start to think hard about how do you analyze networks? How do you think about this problem of interference, where mutations in one region can have disparate effects in other regions? That is going to lead to interesting understandings of mechanisms.
How have advancements in AI impacted treatment approvals?
Machine learning has started to inform FDA treatment rules, either directly through the use of an algorithm as a screening tool or indirectly based on insights derived from machine learning. Some of the algorithms that have been approved are used in a cardiology and radiology context. In cancer immunotherapy, we have algorithms that can predict your mutation burden and the immunogenicity of these mutations. Some of these could be used as screening tools. Based on these outputs, you might be approved for certain kinds of immunotherapy.
Hopefully, these treatment rules just get better and better as our understanding of the mechanisms improve, because the FDA is going to approve based on some mechanistic and causal understanding. Despite some of these screening tools being neural networks, there's still some kind of underlying mechanism that we're trying to understand with them. Is there a binding going on? Is activation of your immune genes going on? At least within the field of medicine, better understanding of mechanisms will lead to better screening tools. That’s my hope.
How has AI affected how you look at data? Has it opened your mind to new possibilities in your own work?
There's always the fear of are you even looking at the right data? Just because you have an enormous amount of data doesn't mean you'll find the answer to the question you're looking for. There have been times where I've wondered do we even have the right kind of data to answer the question we're thinking about? That goes against the zeitgeist of big data: The larger your data set, the more likely you are to find the answers you’re looking for.
In causal inference, we think a lot about, can we even answer the question we're looking to answer? That's a whole subfield called identification, where we're the naysayers. We have theorems that say even if you have infinite data, if it's not the right kind of data, even the most complex machine learning algorithm is not going to be able to learn anything about what you want to know. The nice thing about causality is that you can get domain experts on board more easily because it's much more intuitive. A lot of it is drawn in terms of pictures and causal diagrams where A is pointing to B or B is pointing to A, and so on.
There's always a lot of talk about, where do humans fit in this loop of AI? I'm a machine learning expert. I'm a causal inference expert. But there are doctors out there who know a heck of a lot more than I do about the human genome and cancer. It would be a shame if the algorithms we’re building aren't able to use their knowledge and have them inform what should be the inputs to the algorithm. They can say, “I try to look for patterns in this kind of data.” There's sort of a hunch if this stuff is useful. So maybe machines should also be looking at similar kinds of things, trying to mimic the process of how a doctor is thinking.
Looking ahead, how might discoveries related to causal inference reduce busy work in fields beyond healthcare?
Causal inference has applications across the empirical and social sciences. In economics, for example, we sometimes try to determine the effect of various policies or programs through small scale randomized controlled trials. These sorts of trials often aren’t ideal. They can disadvantage groups that do not receive the treatment, especially when there’s some prior belief that the new program has some benefit, e.g., a new job training program for folks experiencing homelessness. Causal inference methods can help us compute and gain causal insights about policy decisions from observational data and reduce our reliance on conducting such trials.