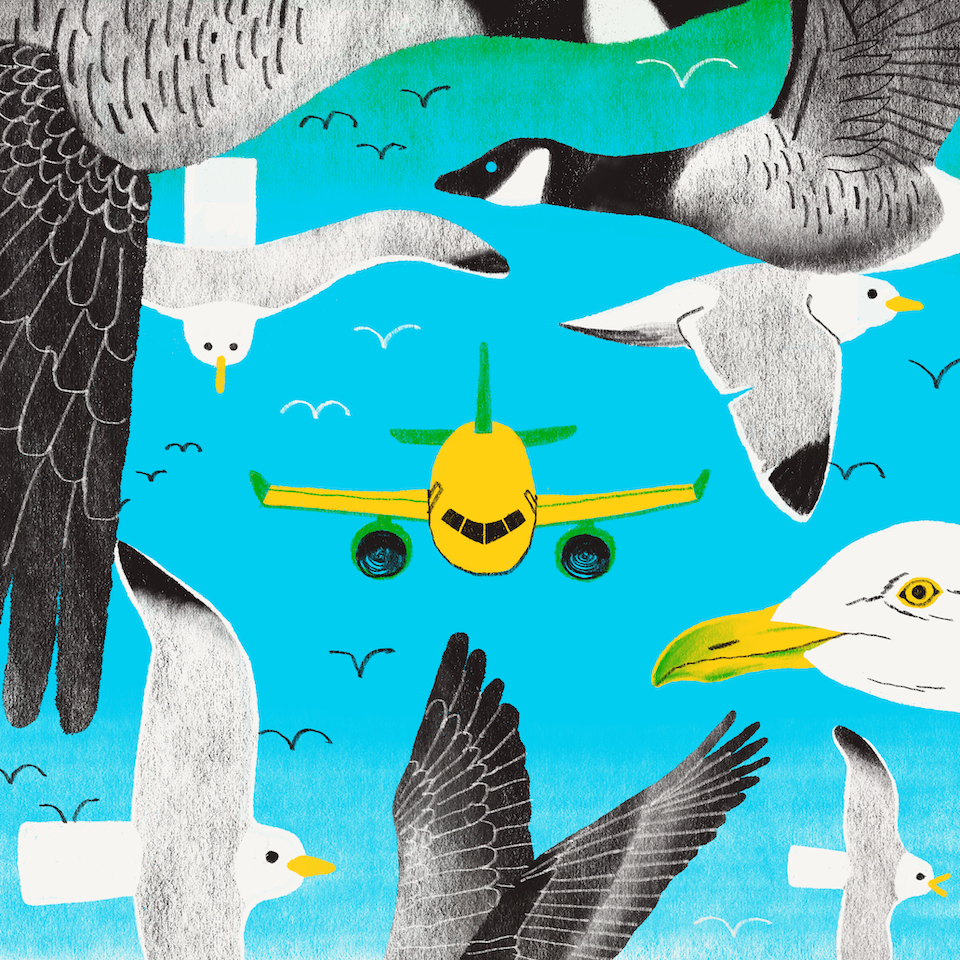
How AI could make air travel safer, smarter, and smoother
Published on August 29, 2024
For air traffic controllers, AI’s potential to predict bird strikes and aircraft collisions could change the game.
Minutes after Captain Chesley Sullenberger piloted the Airbus A320's departure from LaGuardia Airport on January 15, 2009, it started going down. The problem? A flock of Canada geese collided head-on with the plane, knocking out the turbofans of both engines and causing them to lose all power. Sullenberger and co-pilot Jeffrey Skiles managed to glide the plane to a landing in the Hudson River, saving the lives of everyone aboard. Later famously known as the Miracle on the Hudson, the entire incident put into stark focus one of the perils of modern air travel: bird strikes.
Every day, over 45,000 flights navigate the U.S. skies, but during critical phases like takeoff and landing, aircraft are especially vulnerable to hazards such as wildlife collisions. “Bird strike risk is the highest at altitudes from sea level up to a thousand feet,” says Syed Shihab, an assistant professor in Kent State's College of Aeronautics and Engineering. Indeed, in the last three decades, Canada geese smashed into more than 2,100 passenger and commercial flights in the U.S.
While bird strikes rarely lead to catastrophic engine failures, when they do, a miraculous outcome like the one on the Hudson River is hardly guaranteed. Since 1988, wildlife strikes, most of them by birds, have destroyed more than 350 civilian and military airplanes worldwide, killing 491 people in the process.
But what if we could predict bird strikes before they ever happen? With the machine learning tools of artificial intelligence, researchers are betting that they can figure out where birds fly—and if we know where birds are in the sky, we can reroute air traffic to stop potentially deadly collisions. As the aviation industry continues to explore AI's potential, these advancements could change the way we navigate the skies and make air travel safer for both passengers and wildlife.
Predicting bird paths
Whereas some people view bird strikes as just an unfortunate hazard of air travel, to Shihab, the unfortunate predicament seemed like an opportunity for innovation. Along with colleague Elaheh Sabziyan Varnousfaderani, a doctoral candidate at Kent State studying AI-based algorithms for air mobility, they had an idea: Could they build a deep-learning model to learn how different birds move, and then try to predict the bird movement over the next 60 seconds? With such information, it could be a way to not only predict whether a collision will happen, but also go a step further and actively change the circumstances in the air to minimize its chances.
“Bird strike risk is the highest at altitudes from sea level up to a thousand feet."
“If the aircraft still hasn’t taken off, we can delay the departure. If it is already airborne, we can change its heading or climb rate to reduce the likelihood of that collision,” he says.
Shihab chose to focus on pigeons for his research due to their significant involvement in airborne collisions, alongside Canada geese and the European starling. Additionally, a publicly available dataset on pigeon movement near airports facilitated his research. The data itself was gathered from weather and avian radars that detect and track bird movement, and was organized into four columns: time, latitude, longitude, and altitude. With that in hand, he had the entire flight path, in four dimensions, of the average pigeon.
Next came training the AI model to predict the bird’s movement. For that, he turned to an LSTM, which stands for short-term memory neural network, commonly used for any type of forecasting—stock prices, inclement weather—that involves a time series. “You know what has happened in the past,” Shihab explains. “Now you want to predict what’s going to happen in the foreseeable future.”
Using an LSTM built with the Python programming language, he trained the model by feeding it the inputs from the public data set. Over time, the neural network learned exactly how a pigeon moves and at what velocity. It learned the bird’s maximum altitude, as well as how pigeons turn in air and adjust their heading. Shihab took that network and applied it against simulated aircraft departures from nearby Cleveland Hopkins Airport, and found the LSTM was able to predict the flight track of a pigeon within 100 meters of an airplane at take off, and with 95 percent accuracy. He and Varnousfaderani published and presented the data last year at the AIAA Aviation and Aeronautics Forum.
Currently, airports rely on inspecting bird remains and employing scare tactics to prevent strikes. Crews dispatched by the U.S. Department of Agriculture will set up pyrotechnics, cheap fireworks, and even deploy trained agents with shotguns to keep birds at bay. If AI models like the one Shihab developed could be incorporated into modern air travel, it could flip the script, making technological innovation the new instrument to protect birds, pilots, and passengers.
Making air travel safer and easier
The AI methods developed by Shihab and Varnousfaderani have broader implications beyond just bird traffic when it comes to managing the skies.
“The same approach for preventing bird strikes can also be used for preventing aircraft-to-aircraft collisions,” says Shihab. “Based on their flight plans, we can use AI to determine the optimal way of avoiding collision between two or more aircraft.”
At the University of California, Merced, Ayush Pandey is researching how AI might help the 14,000 air traffic controllers employed by the Federal Aviation Administration. Pandey is an assistant teaching professor of electrical engineering with a background in dynamical systems and control. His current project considers how generative AI models can help parse the reams of text-based data that’s collected as pilots and tower operators communicate back and forth, controlling an airplane’s flight path upon take off, in the air, and during landing.
“That’s fertile ground for generative AI to step in,” he says. “You could input whatever communication is happening, and then increase the efficiency of some of the decisions that are being made.”
“If the aircraft still hasn’t taken off, we can delay the departure. If it is already airborne, we can change its heading or climb rate to reduce the likelihood of that collision."
Say an air traffic controller orders a plane to deviate for a thunderstorm or turbulence up ahead. An AI model, over time, can construct a pattern based on each time such a command is given. Eventually, the model will be able to predict when and where a plane should change course, effectively acting as a backup to confirm, or alter, the decisions being made between the controller on the ground and the pilot in the air.
Flight delays can also be tackled using AI. At London Heathrow Airport, a current trial makes use of HD cameras and machine learning to boost the airport’s landing capacity during periods of low visibility. Although the control tower at Heathrow is impressively tall at 285 feet, it can nonetheless disappear during periods of low cloud coverage, obscuring views and making it difficult for air traffic controllers to clear planes for takeoff.
By using AI technology, the airport can pull in images from 20 separate HD cameras deployed to the airfield, interpret the images, track the aircraft, and then tell those in the tower when a plane has departed. Knowing which runways are clear allows controllers to guide incoming planes to a safe landing—not to mention knowing which runways can launch a plane waiting in the queue. With real-time information on runway availability coming in, traffic controllers don’t have to depend on good weather conditions and zero cloud coverage to see, literally, where passenger planes are located on the airfield.
The future of air travel
Right now, the sorts of use cases being discussed and researched by Shihab and Pandey are in their theoretical stages. In the US, the FAA is taking a cautious approach to how AI might be used to improve safety. An AI roadmap, outlining the certification framework for how commercial, military, and even space flights might make use of new machine learning technologies, is still forthcoming. As Shihab points out, the data required to train AI models for air traffic must be comprehensive and robust: one missed data point could spell disaster for a plane relying on an AI application to change its flight path.
Still, chances are that AI will become more and more useful to the air industry. Much in the same way that AI models are used to train the navigation systems of self-driving cars, so, too, will AI become a useful tool for aircrafts when it comes to improving air travel—not only for the planes, but for our feathered brethren as well.
“AI is being applied at a research level,” says Pandey. “But it will definitely be integrated more and more with our air traffic navigation.”