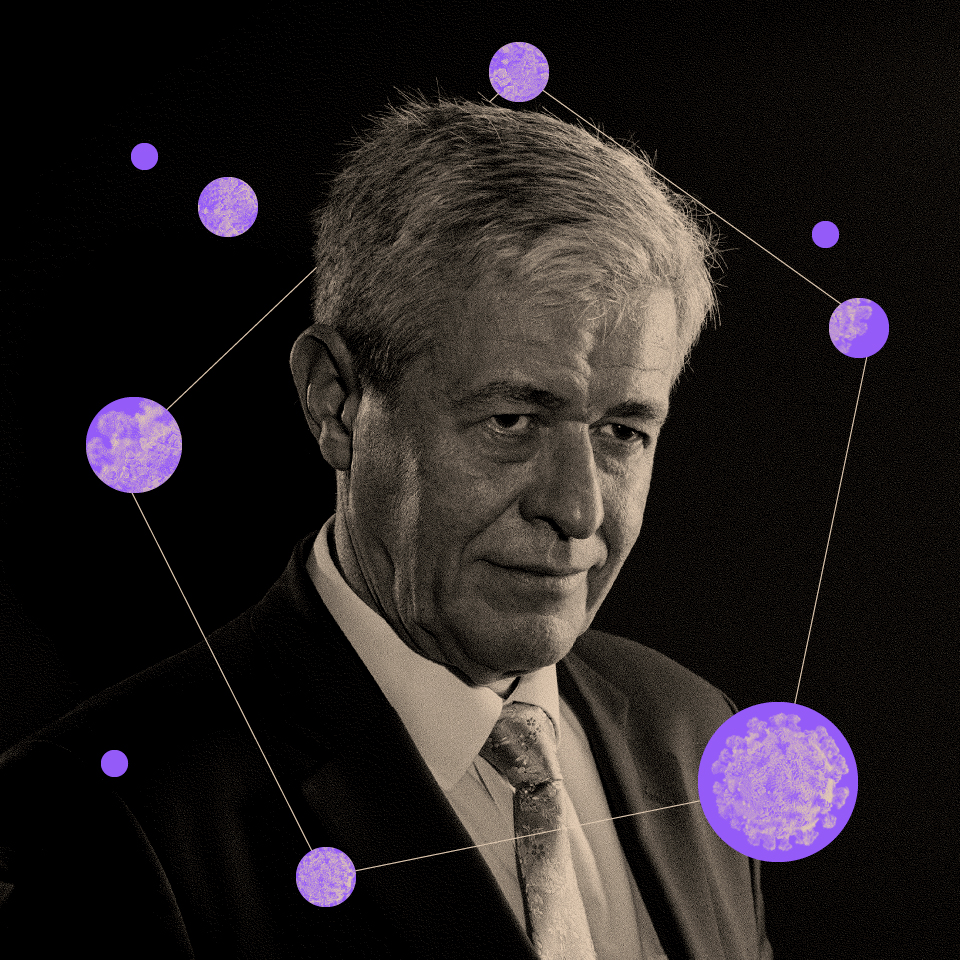
or, how to model complexity (lots of it) by facing your uncertainty
The lockdown had begun in London but for neuroscientist Karl Friston, it was just an average day. He had just met with his neurobiological modeling group as he does on late Monday afternoons. Friston is a creature of habit, and finds disruptions of routine anxiety-provoking.
But the fact that this group had only just begun meeting by video conference, that this afternoon’s meeting was twice the normal size, and that the modeling was about a viral epidemic and not human brains, doesn’t seems to have troubled him. “There is novelty here, and you respond scientifically,” he says, “as a good scientist you just practice your profession in this new context. The whole point of being a scientist is that you're always on the search for the resolution of uncertainty. You're always going out there, trying to find those data, those experiences that no one has ever had before. So this, from my point of view, is just an average day.”
Friston is the most widely cited neuroscientist in the world, but hardly a fixture in epidemiology. His contributions to brain imaging have revolutionized the field, and also revealed the elegant mathematics that underlie how the brain does what it does. The reason for his relevance in the present moment is not just whether his group’s model of COVID-19 will turn out to be useful to policy makers, but if his method of thinking is uniquely suited to what we now understand as 21st century problems. Our existing mental models for things like epidemics are still shaped by the 1854 Broad Street cholera outbreak in London that was traced to the water from a single well. It turned out to be a very linear problem. John Snow, who founded the field of epidemiology, stopped the incidence of new cases by removing the pump handle.
21st century problems, by contrast, are wickedly non-linear. They have many degrees of uncertainty that combine to produce catastrophically surprising effects. Global climate instability, financial market volatility, and the present pandemic are all examples of systems that are impossible to reason about in linear terms—there is no pump handle to remove.
Brains have evolved to make sense of the kinds of uncertainty we’re facing now on a mass-scale.
Scientists and medical practitioners are working tirelessly to battle the current crisis, and Friston has been applying what he’s learned by modeling brains to the pandemic. Brains have evolved to make sense of the kinds of uncertainty we’re facing now on a mass-scale. Every brain learns to be a brain. It follows some ancestral phylogeny to become the brain it currently is. To a baby, the world is a very uncertain place, and the mother represents certainty and their first teacher. We learn to depend on our parents, families, and extended community as models that tell us how we should behave. Through practicing these human social behaviors we become autonomous humans ourselves.
Think about how amazing this is. Think of the complexity of the problems facing a young child that they don’t have the mental scaffolding to understand. And then, one day, they do! These are breakthroughs in learning: We know how to learn things that we don’t yet have the mental structure to understand. The brain does this by building models of the world that generate behavior appropriate to the world its models assume, and then looking for evidence that proves those assumptions.
This is how Karl Friston is proposing we try to understand the COVID-19 pandemic. Like the fledgling brain, we have to build models that capture the uncertainties we confront. And like the brain, Friston believes these models need only be complex enough to limit our major uncertainties at the moment. The way we, and our brains, achieve more precision is not through adding more complexity, but in networking these rough models together in ensembles and hierarchies that constrain each other.
The goal now for Friston and his eclectic and international band of collaborators is to use their methods to help create the measure of scientific confidence that will allow us to safely resume moving about our cities and towns. Modeling uncertainty doesn’t sound terribly promising. Isn’t the idea to get rid of uncertainty, especially in times like these?
Facing uncertainty
“If you don't represent your uncertainty, you cannot define the complexity,” says Friston. A global pandemic is a complex problem. There are lots of data to consider, and the challenge is to know which are most predictive. But this pandemic is a particularly uncertain phenomenon. As it moved from east to west, it affected neighboring countries quite differently. And many infected with the virus appear completely asymptomatic while others are quickly overcome with horrific and often fatal symptoms. How do you model something that presents more questions than answers?
The brain, like a scientist, is also always searching for the resolution of uncertainty—and the world provides an unlimited supply. To explain how we keep from being overwhelmed by all of the evidence of our many senses, Friston has identified two useful concepts. The first is the free energy principle which unifies perception and action as a dynamic tradeoff. At any moment, Friston asserts, there’s an optimal balance between paying attention to sensory information, like noticing a car speeding towards you, and executing an action, like swerving out of the way. The free energy principle says that the brain will recruit the resources necessary to reduce its uncertainty over any given period of time. Of course, the world is a very uncertain place, and whatever time frame our driver was operating on previously, it compressed immediately with the velocity of the oncoming vehicle.
The second concept is one Friston borrowed from AI pioneer Judea Pearl called the Markov blanket. All living things require boundaries to survive, and the Markov blanket is a mathematical definition of that boundary. In a sense, your car is your active boundary moving through space, and your attention is your perceptual boundary. Your job as a driver is to preserve those boundaries and avoid crashing into that car.
Putting these two concepts together has enabled Friston to create models of complex systems that change over time, like brains or pandemics. These models are hierarchical—they have layers—because that’s what complexity is. The Markov blanket that contains you is contained in turn by that of your car, your city, and so on. Just as there are different areas of the the brain that influence each other, geographical COVID hot spots in one city eventually reach the neighboring towns. No country or state can make decisions without taking into account what’s happening with its neighbors.
“The assumption that connectivity is always a good thing is for me so naive.” —Karl Friston
Speeding cars and novel coronaviruses present risks to the preservation of our boundaries. We live now in the jeopardy of a hyper-connected world, accelerated not only by technology and the internet, but through affluence, commerce, and global travel. “The assumption that connectivity is always a good thing is for me so naive,” Friston says. “From the point of view of that delicate self-organization that enables these Markov blankets that constitute ourselves, or a society, or an ecosystem to survive, connectivity is the killer,” he explains. “It's basically putting energy into a system and literally boiling it—and destroying all that delicate structure.” Life depends on maintaining boundaries, and deadly viruses find ways to breach them.
Today’s pandemic, like the brain, is dynamically unstable. Twenty years ago, Friston demonstrated that dynamic instability was “crucial for adaptive brain function.” In the time since, the study of complex systems has shown that this instability generates the diversity on which selection pressures act to produce adaptive behavior. In other words, we need disorder to learn, even though it’s stressful.
Adaptation, for Friston, has a very precise definition. It is the most energy efficient way to accomplish some action, over the set of systems engaged in that action. This free energy minimum is also an information maximum. Imagine you have to go out and take pictures of landmarks in a city. The path that is the shortest distance between all of the points is also the one where you’ll take the most pictures in the shortest time.
In free energy terms, the brain is in a hurry and finds the simplest model that’s accurate enough. The everyday conscious mind operates on a beat of about 100ms. Under conditions of uncertainty, not getting information in time is the same as getting the wrong information. Your brain, Friston says, must “aspire to provide accurate accounts and responses to any data that you're confronted with, while at the same time minimizing the complexity, so that you can generalize to the next wave of new information that comes along.”
Modeling complexity
Bringing this back to modeling COVID-19, Friston’s gambit is that the way brains predict what will happen in the world is analogous to scientists trying to generate accurate data for infection and mortality rates in the future. These models do not produce predictions, but rather simulations or projections with very high statistical accuracy. The key is to use historical data to set the parameters within which a model generates forward projections. Predictions based solely on fitting curves to historical data, Friston believes, can never account for the full variability of what happens in the world.
Two weeks after I spoke to him, Friston’s group published a paper describing the dynamic causal model of the virus’s spread in the UK. The complexity of the model is similar to what Friston would use to describe the behavior of a population of neurons in the brain’s visual cortex in response to some stimulus originating in the retina. But instead of neurons and milliseconds, the COVID model substitutes people and days.
These population dynamics, whether expressed as people or neurons, take the form of what Friston calls “transients.” Just as a transient is a stranger in town, someone just passing through, a transient in the brain is a detectible anomaly amid routine neuronal behavior. So if your eye sees a flash of light in some part of your visual field, a brain scan will register a transient response in the visual cortex some number of milliseconds later. It will ramp quickly and then decay. It will have a characteristic shape, a curve that spikes or flattens.
These transients have some interesting properties that make them good stand-ins for real world events like pandemics. Their shape can affect activity in multiple brain locations in an identifiable way. Although these effects can be synchronous, and even cyclical, they are most often asynchronous, because things take time to move through space. Just as the outbreak in Italy preceded the one in France, the message from the retina, which is the transient, may be expressed in one region of the brain before another.
fMRI brain scans have long been able to identify synchronous effects, supporting Donald Hebb’s now famous mid-century edict, “cells that fire together, wire together.” But Friston’s groundbreaking work at the turn of this century on the importance of asynchronous transient effects opened up new ways to model the uncertainty of things that don’t all happen at the same time. His theory of active inference suggests that the brain is in the game of efficiently summarizing statistical representations of uncertainty so that it can keep up with the relentless demands of sensory stimuli and the need to act quickly and effectively in the world.
In these terms, we can conceive of the world as a complex dynamic system like a brain, and COVID-19 as a discernible ripple in that system. Those of us who follow the Johns Hopkins dashboard of COVID-19 statistics experience the asynchronous effects on the shape of the flattening curve when new testing data updates the height of previous days’ bars.
Without getting too deep into the weeds, it’s worth looking at Friston’s dynamic causal model (DCM) of COVID-19 in a little bit of detail to see the kind of thinking involved in understanding these uncertain effects. The model is an augmented version of a classic SEIR model from epidemiology. The letters stand for Susceptible, Exposed, Infectious, and Recovered, which are the four states of infection that a person can be in.
In the Friston model, infection itself is one of four factors, along with location, clinical status, and testing status, each of which can be in one of four states for each person in a population. The important point here is that to write such a model you have to define your uncertainty: What are the most relevant questions to ask about the status of the population each day? The Friston group determined those questions to be where each person is, whether they are infected, whether they have symptoms, and whether they have been tested.
By putting these questions into a diagrammatic form (see figure below), you can express the causal relationships between the parts of the model. One can go back and forth from home to work, but if you go to the CCU (critical care unit) your next step is either back home or to the morgue. Similarly, if you develop severe symptoms of ARDS (acute respiratory distress syndrome) your ability to go to the CCU is limited by the availability of beds. And the death rate of people with ARDS who stay at home is tragically higher than those who get the proper care. You can write all these kinds of details into this generative model, which creates a precise enough representation to be statistically accurate.
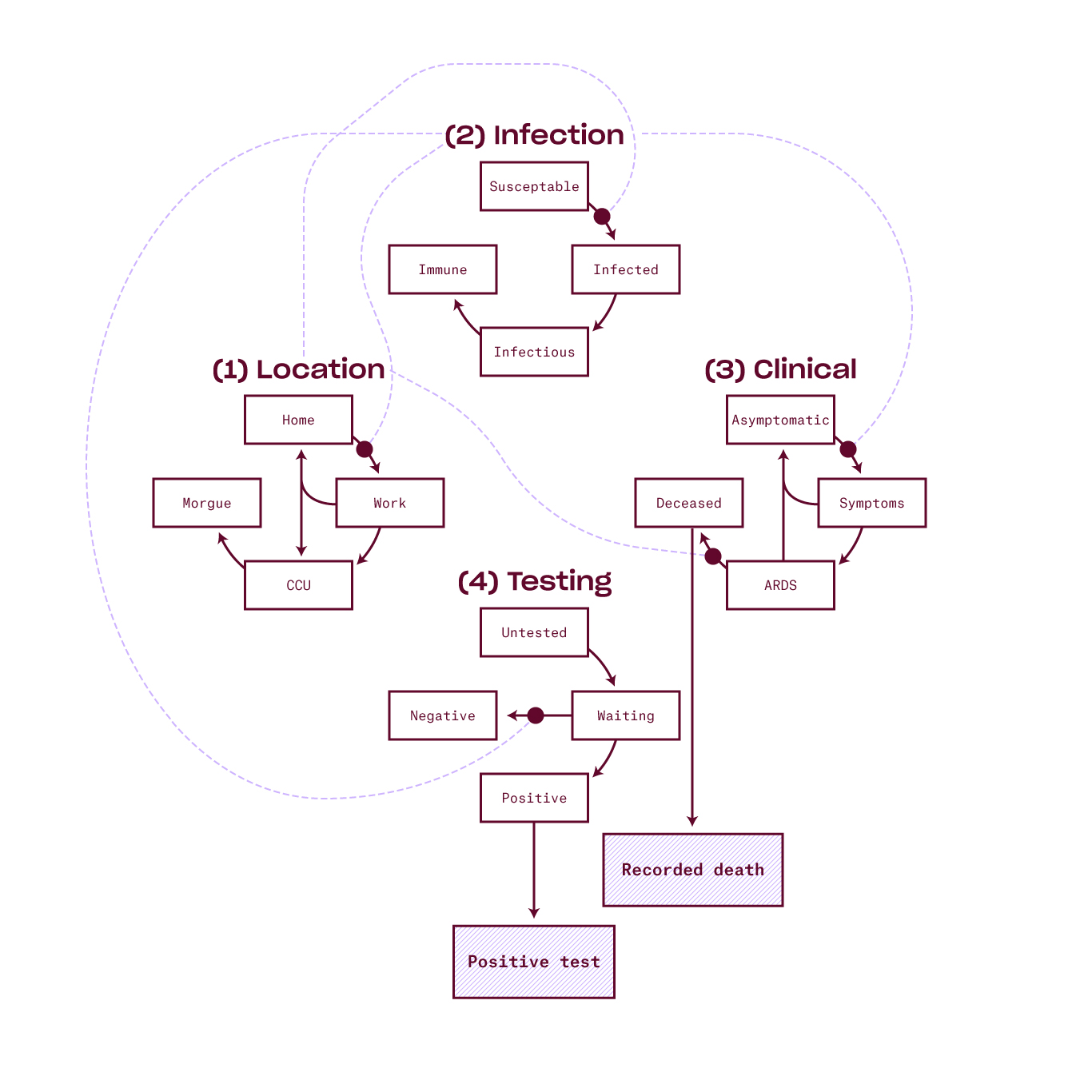
Just laying out the questions that define a system, and working out the dependencies between the answers, actually tells you quite a lot. In this particular case, the data to estimate are the number of recorded deaths and the number of positive tests each day. The model operates within parameters that represent a given population in a certain place. These include things like the size of the effected population, the number of people infected, and the infection rate, all at the beginning of the time period represented by your model.
In a standard SEIR model, there are many levels of uncertainty behind the number of people in each state. How susceptible a person is relates to how many people they come in contact with (determined by their location). How many people are actually infected has a lot to do with whether infected people are symptomatic, and what percentage have been tested. Infectiousness itself is higher if asymptomatic people can spread the virus, as is the case with COVID-19. And finally, even immunity after infection is not a foregone conclusion.
Reclaiming causality
The most important difference between the DCM model and almost all of the hundreds of others contributed by teams around the world is in the “causal” part of the name. Everyone has access to the same publicly available data sets, but a causal model tries to understand the reasons behind how that data was generated. Let’s say a state reports 100 COVID-related deaths, but only counts deaths occurring in hospitals. Their death reporting policy could be a reason why the data is underreporting the true number of deaths caused by COVID.
To look at this another way, a pandemic is not a single, monolithic event. It is a series of localized epidemics, each with their own history, shaped by different policies, that generate a series of data. You can use that local data to project its future trajectory given the local policies. But what you can’t accurately do is use that data to predict the death toll in a different place with different demographics and different policies. Only by addressing the underlying variations that generated the data—with explicit parameters that reflect the local conditions—can you begin to extrapolate data from Italy, for instance, to the UK. And this is precisely what Friston’s group has done.
The initial results of the Friston model correctly projected that London would be able to keep the infection rate below the carrying capacity of the National Health Service, but also made it clear how far from herd immunity the UK will be by the end of this first wave of infections. A comparative analysis of this model applied to seven countries in Europe done by Friston’s colleague Rosalyn Moran concludes that only 6% of the population in these countries will be immune after these initial outbreaks. This contrasts with the 60% of a population most epidemiologists agree is required for herd immunity effects to take hold.
“We’re imagining that a worldwide pandemic is composed of these regional outbreaks, much like a chain of dominoes.” —Karl Friston
Friston may not be getting back to brains any time soon. A couple of weeks after the first paper, his modeling group released a second to show how the state of infection in one location affects its neighbors. In this case they modeled eight US states to show how increased mobility when social distancing is relaxed could lead to a second wave of infections. “We’re imagining that a worldwide pandemic is composed of these regional outbreaks,” says Friston, “much like a chain of dominoes would cause each other to fall in succession, where each domino can be likened conceptually to the episode that we’re trying to model.” He hopes this more sophisticated sort of model will be valuable as we move into the next phase of the crisis. Accounting for our uncertainty is crucial as we collectively decide how to resume normal life, and when.
If you follow Friston to his logical conclusion (and he’s always impeccably logical), this approach to facing uncertainty is applicable to everything. Whatever you’re trying to figure out is a system bounded by the answers to certain questions. In our daily lives we follow this same pattern, and it’s even more poignant now during lockdown. We go out into the world looking for answers to reduce our uncertainty. What is it like outside your house? When you walk by people on the street, do they make space for you or barrel on through without looking? Is the world filled with people like you, whose behavior you can accurately predict based on your own? Or do you need to be vigilant, because you’re uncertain about what you will encounter?
What’s important is to ask the questions at the right scale. In terms of how I feel social distancing on the street, the questions are at an individual level. But to model an epidemic, you ask questions about a population. “At a higher level,” Friston says, “we can just dissolve the distinctions between you and me. Just under the assumption that we all are the same, and model the actual spread of virus and infections as if it was an explosion, literally a fire, percolating through an ensemble of human atoms under certain constraints.” He takes a breath, and sighs. “So my life has changed in no way whatsoever, in terms of its themes and the kinds of things I'm doing, but the content has changed, and is changing rapidly.”